Leveraging AI to Level Up Network Management
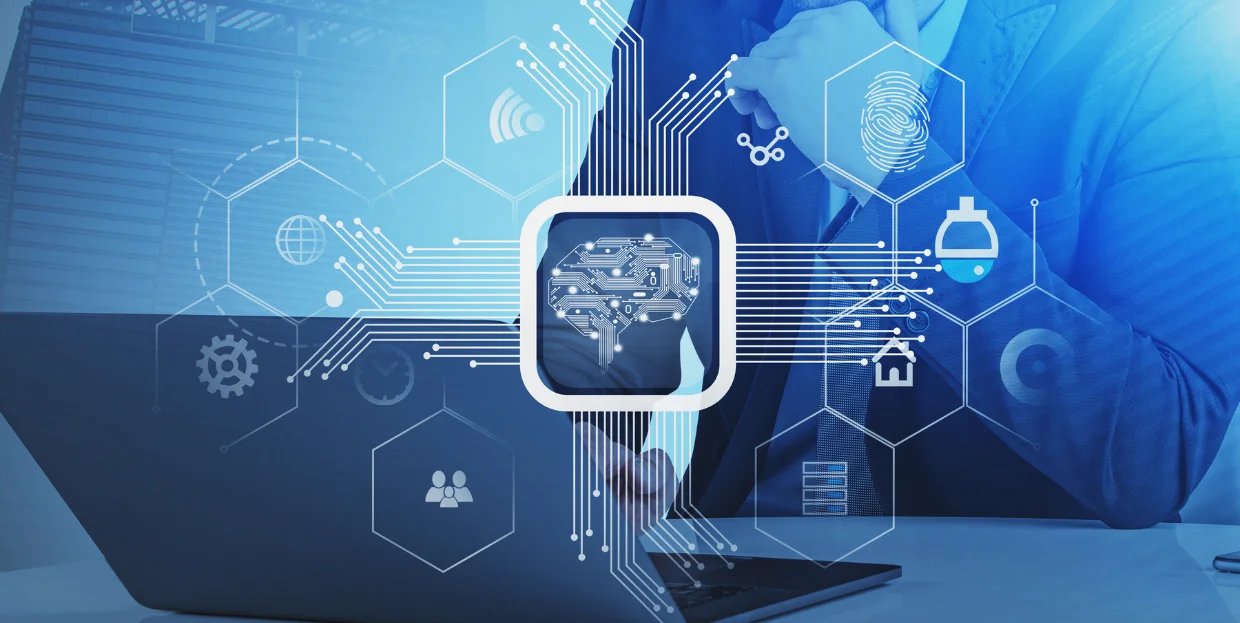
Introduction
With network management becoming increasingly complex in the modern digital landscape, organizations are searching for new processes to simplify their network operations. The growing emphasis on cloud and hybrid IT solutions is a challenge for IT teams to keep up with. This innovation, coupled with inexperienced engineers, means teams often struggle to manage their networks effectively.
Businesses are utilizing AI’s growing capabilities to simplify and enhance network operations. Technologies like machine learning can automate repetitive business tasks, scale workloads, and optimize network efficiency.
When utilized properly, AI has the power to transform network management and enhance connectivity around the world.
The Growing Challenges of Network Management
IT developments like hybrid cloud environments and IoT devices contribute to the rising complexity of network infrastructures. As these practices become more common for organizations, data is exchanged across numerous channels and devices, which means security measures must also increase.
These data exchanges present new business challenges, like real-time monitoring, fault detection, and resource optimization. To ensure the safety of your business data, you must continuously monitor for faults and resolve their impacts on your environment.
While IT teams previously handled these challenges independently, the recent spike in cybersecurity threats, coupled with the evolution of network environments, surpasses a team’s capabilities alone. Traditional methods cannot deliver the same results as AI-integrated solutions for dynamic network environments.
AI-Powered Solutions for Smarter Networks
Many organizations are beginning to integrate AI into their network management solutions to assist their IT team and boost productivity. AI technologies like machine learning and deep learning improve efficiency by monitoring networks for anomalies and following human-like decision-making processes to address them.
Currently, AI is used for tasks like analyzing traffic patterns, predicting faults before they occur, and optimizing system configuration. By implementing AI solutions, employees reduce time spent on repetitive tasks, allowing them to focus on improving overall operations. AI is also more accurate in identifying issues than humans.
Ericsson’s research shows that AI can identify 50% more network problems than humans, with almost perfect accuracy. This integration improves business efficiency by as much as 30%.
Key Benefits of AI in Network Management
What are some key benefits of integrating AI into your network management system?
Proactive Issue Detection and Resolution: AI enables predictive maintenance by identifying anomalies before they impact the network, reducing organizational downtime. Businesses like Orange Wholesale, a global telecom company, use AI-driven programs to minimize the mounting costs of system downtime.
Optimized Resource Allocation: Machine learning models dynamically allocate bandwidth based on real-time traffic patterns. This improves service quality for critical business applications.
Improved Decision-Making: AI augments human decision-making by providing actionable insights based on data analysis.
Innovations Driving AI in Network Management
As AI technologies become more advanced, their capabilities are harnessed in new, innovative ways. IT professionals continue exploring use cases for preexisting and emerging AI technologies in network management.
Interactive AI: Some AI models have unique interactive capabilities that allow the technology to adapt to dynamic network conditions and user needs. These programs can interact with users in real time to provide customized recommendations based on their IT problems. Interactive AI models are often seen in smart devices and assisting customers with questions on company websites.
AI-Driven Network Optimization: Self-learning AI models optimize network paths, creating greater efficiency throughout the environment. The team at Ericsson has found that AI optimizations can reduce latency in 5G networks by up to 10 milliseconds.
Practical Applications of AI in Network Management
Figuring out how to incorporate AI into your business structure can be difficult. Here are some examples of how businesses use AI programs to facilitate operations.
Automated Network Monitoring: AI tools analyze traffic data to detect and prevent DDoS attacks to minimize interruptions to a server.
Dynamic Configuration Management: AI systems are automated to adjust network settings and meet performance goals.
Enhanced Security: AI detects and mitigates security threats in real-time, ensuring that networks remain protected against attacks.
Overcoming Challenges in AI Adoption
Integrating AI solutions into your network management system may come with some challenges. Knowing what challenges exist and how to address them will improve the adoption process for your business.
Data Quality and Volume: Though it seems more time-consuming on the front end, your organization should use high-quality, labeled datasets when training AI models. This trains the systems to analyze and interpret business data more effectively, ensuring the accuracy of AI solutions.
Explainability in AI Decisions: One of the most prominent challenges with AI is trusting the results it produces. Without a clear explanation, users are expected to have blind faith in the output of this technology or otherwise spend significant time verifying that results are accurate. For this reason, you should ensure that your AI is transparent and that its actions are interpretable.
Machine Learning Operations Integration: MLOps is critical for maintaining and updating AI systems. It ensures that new machine-learning models are deployed correctly and continuously function as intended, especially as updates and changes are released.
Future of AI in Network Management
What will the future of AI look like? While we don’t know exactly how AI will evolve to meet business needs, we can make predictions for future AI integrations.
With emerging trends like Edge AI, we expect to see real-time processing and more efficient data analysis. Instead of relying on the cloud to store and process data, this can occur on edge devices.
AI’s rapid processing capabilities may also create 6G mobile networks, allowing for global hyper connectivity and smarter network management. Plans for rolling out this technology could start as early as 2030.
Conclusion
As our understanding of AI’s capabilities continues to evolve, we can harness this technology to transform how we manage networks. From automating tasks to proactively monitoring network environments, AI will enhance IT teams’ work to sustain long-term operations.
With new technologies like machine learning and interactive AI, businesses can more effectively meet consumers’ needs while optimizing operations. This will lead to enhanced efficiency and performance within organizations.
Explore how Plow Networks can provide your business with IT services to improve network management and enhance security for long-term success.
Explore more on: